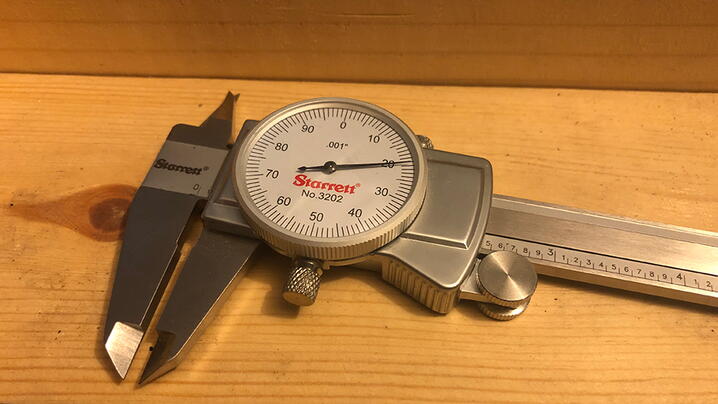
“We should measure impact, not output.”
Across the public and nonprofit sectors, every time I’ve suggested implementing operational reporting as a management tool, I’ve heard this rebuttal. I doubt I’ve ever waited more than 15 seconds to hear it. In local government, this mostly comes up in relation to strategic planning—writing a new strategic plan or tracking progress toward an existing one.
Output looks inward, at operations. As an example of output measurement, a city may count the feet of bicycle lanes it builds. Impact looks outward, at society. As an example of impact measurement, the city may track the growth in bicycle ridership.
It’s common sense that impact is what ultimately matters; a badly routed bicycle lane might be worthless. Unfortunately, impact is slow to appear and hard to measure. It depends on the same messy internal data that powers output measurement. I’ve found that measuring output with precision and regularity is practically a prerequisite to trustworthy impact measurement.
Impact measurement is slow
Impact can be slow to appear because services can take a long time to soak in and change lives. A bicycle lane might take years to achieve full impact as residents’ habits and possessions evolve to use it. That’s in addition to the time needed for analysis, so the lead time for impact measurement is generally long regardless of the tools at your disposal.
Contrast this with counting the feet of bicycle lane. Perhaps two construction companies are working on bicycle lanes, and you find that one is moving three times faster than the other. Is the faster vendor cutting corners, or is it more efficient? (Both? Neither?) Following up could deliver vital value for residents, days or weeks after work commences—if good output measurement infrastructure is in place.
Impact measurement is hard
In the meetings where it’s suggested to measure impact and not output, the idea is to run a survey before and after service delivery. The change in the results is the impact. Easy, right?
This doesn’t work because the world changes and doesn’t look the same everywhere.
If the world were perfectly static, the simple survey idea could work. But sometimes a professional cyclist becomes a national hero, and everyone buys a bicycle; sometimes they fall into scandal, and the bicycles start collecting dust.
I’m often asked to analyze survey data after the fact. The results of analysis are typically useless because the service-related impacts are swamped by external changes—sometimes, to the point that metrics move in the opposite direction as was hoped.
Similarly, if the world were perfectly homogeneous, you could simply compare your residents, who get your services, with nonresidents who get different services. But that’s not true, either—people live where they do precisely because they are different.
Randomized experiments, akin to clinical trials for new medicines, are the textbook solution. For some services, one can randomly assign some residents to a wait list while helping the rest, or award a scarce service by lottery. Randomization provides a clean basis for comparing outcomes, but withholding services isn’t always an option, legally or ethically. (And some assets, like bicycle lanes, are public infrastructure that’s available to all residents or none.)
Another strategy is to statistically divine an apples-to-apples comparison within observational data. With intricate “causal inference” techniques, one takes advantage of historical irregularities—like program rollouts or natural disasters—to sniff out impact without the luxury of experiments. These tools require extraordinary care to give anything but wrong answers.
I work on causal inference only occasionally, so I talked to someone who does it every day. Trang Thu Tran is a senior economist at the World Bank. The research component of a Ph.D. in applied microeconomics like hers—several hard, full-time years by a brilliant mind—often consists of one or two good impact measurements. And even with 10 years of subsequent experience, Tran sees a limit to how much faster the work gets: “I typically don’t see any credible, robust program evaluations that take less than quite a few months,” she said. “On a regular basis—day to day, year to year operations—I don’t think it’s feasible” to measure impact.
Data quality matters
Finally, I often hear that output measurement is so easy that it should be settled at junior levels, leaving elected officials and senior management to focus on strategy and impact. From my decade in the data sausage factory, I suggest that output measurement is seldom too easy and a great way for senior leaders to grow into a data-driven style.
It’s hard to describe how nasty operational data tend to be. Sometimes, due to missing or inaccessible data, performance measurement is impossible but winds up in a city or county’s strategic plan (and budget) because nobody knew.
When humans enter data, there will be typos. Rookies will enter data incorrectly as they learn systems. When machines generate data, there will often be anomalies and no person to explain them. The format or meaning of data will change mid-flight due to software changes beyond your control.
Output measurement can serve as a canary in this coal mine. When a management analyst familiar with day-to-day operations reviews organizational output (in a report or dashboard), they naturally notice things that look “off.” Such intuition, applied routinely, is what makes data quality improvement tractable.
Attempting to skip this routine output measurement for a straight shot at loftier impact measurement, the data challenges worsen. Problems with data collection ride indefinitely instead of being fixed, so data availability and data quality deteriorate. Operational anomalies in the record—juicy anomalies like service disruptions that give causal inference tools something to bite into—float off into distant memory. These retrospective challenges are typical of Tran’s research. She reports that they are “what I grapple with all day. It’s a data quality issue. Is the data that you see actually measuring what you wanted?”
Simply put, consistent senior engagement with organizational output throws sunlight—the best disinfectant—on internal data.
Conclusion
“What would the world be like if it weren’t for our work?” The impact question, probing deeply at one’s role in society, demands honest answers. Built from difficult quantitative inquiry with a long lead time, the answers rely on internal data that usually need whipping into shape.
Consider measuring output first: “How much did we produce last month compared to a year ago?” A habit of answering such questions will move you closer to answering the impact question while delivering better services for residents along the way.
Find about Performance Management from ICMA. Or download our Strategic Planning in Small Communities: A Manager's Manual
New, Reduced Membership Dues
A new, reduced dues rate is available for CAOs/ACAOs, along with additional discounts for those in smaller communities, has been implemented. Learn more and be sure to join or renew today!